Latest Updates in Machine Learning: Navigating the Future of AI
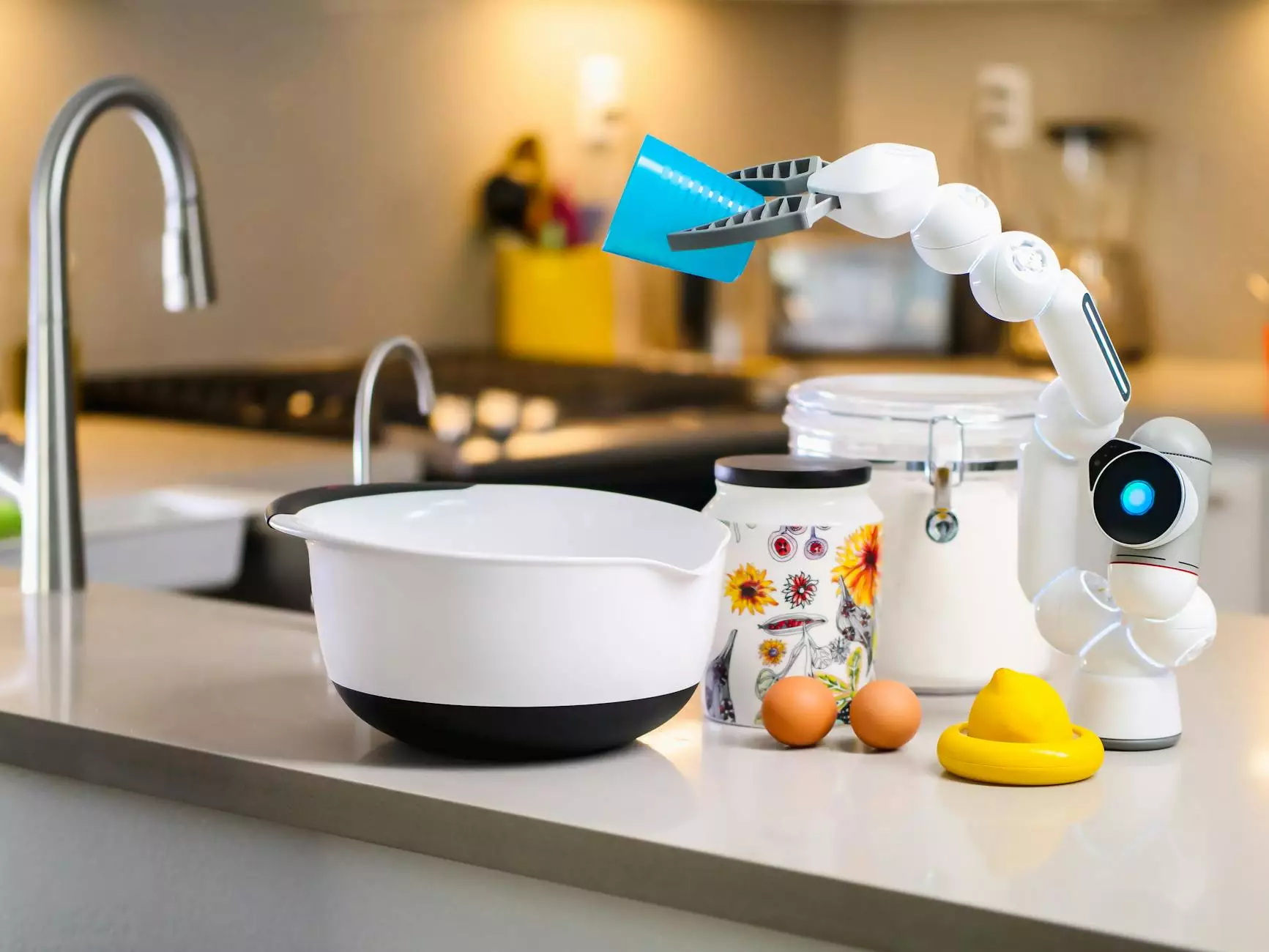
As we journey through the 21st century, technology continues to evolve at a breakneck pace. Among the various innovations, machine learning stands out as a powerful tool reshaping both industries and daily life. This article delves deep into the latest updates in machine learning, examining groundbreaking advancements, trends, and their implications for businesses and society at large.
Understanding Machine Learning
Before diving into the latest updates, it's essential to understand what machine learning (ML) is. Machine learning is a subset of artificial intelligence (AI) that enables systems to learn from data, identify patterns, and make decisions without being explicitly programmed. This capability opens a world of possibilities for automation and predictive analytics, making ML a focal point for innovation.
The Core Components of Machine Learning
Machine learning algorithms typically fall into three categories:
- Supervised Learning: Involves training a model on a labeled dataset, where the outcome is known. The model learns to predict outcomes based on input data.
- Unsupervised Learning: This approach deals with unlabeled data, allowing the model to find hidden patterns or intrinsic structures in the data.
- Reinforcement Learning: Here, an agent learns to make decisions by taking actions in an environment to maximize some notion of cumulative reward.
Recent Breakthroughs in Machine Learning Technology
The field of machine learning is currently experiencing unprecedented growth, with numerous breakthroughs that shape its trajectory. Let's explore some of the most notable latest updates in machine learning.
1. Enhanced Natural Language Processing (NLP)
Natural Language Processing has made significant strides, primarily due to advancements in deep learning models. The introduction of transformers, particularly the BERT and GPT models, has revolutionized the way machines understand and generate human language. These models allow for:
- Improved sentiment analysis
- More coherent text generation
- Advanced translation services
Businesses leveraging NLP can enhance customer support, produce content, and interpret vast amounts of textual data more effectively.
2. Automated Machine Learning (AutoML)
AutoML tools are simplifying the process of deploying machine learning models, making them more accessible to non-experts. These tools automate tasks such as feature engineering, model selection, and hyperparameter tuning. As a result, organizations can:
- Reduce the time and resources needed for model development
- Encourage collaboration between data scientists and domain experts
- Facilitate rapid experimentation and prototyping
The rise of AutoML is paving the way for wider adoption of machine learning across various sectors, driving innovation.
3. Federated Learning
As privacy concerns become more pressing, federated learning has emerged as a solution that allows models to be trained across decentralized devices with minimal data transmission. This approach ensures data privacy while still leveraging insights from diverse data sources. Key benefits include:
- Maintaining personal data on user devices
- Building robust models without compromising privacy
- Enhancing collaboration across organizations while respecting data regulations
4. Explainable AI (XAI)
With machine learning algorithms gaining influence in decision-making, the need for transparency and accountability has never been greater. Explainable AI focuses on developing models that are interpretable and understandable by humans. This is crucial for:
- Building trust with users and stakeholders
- Ensuring compliance with regulations
- Facilitating better decision-making processes
XAI methods not only clarify how models function but also help identify biases in training data, making machine learning fairer and more reliable.
5. Advances in Computer Vision
Computer vision has seen remarkable advancements, driven by deep learning techniques. Recent updates include:
- Improved object detection algorithms
- Enhanced image segmentation capabilities
- Real-time video analysis systems
These advancements are being utilized in various fields, including healthcare, automotive, and security, enhancing efficiency and accuracy.
Challenges Facing the Machine Learning Landscape
Despite the exciting updates, several challenges persist in the machine learning domain. Addressing these challenges is vital for the continued growth and acceptance of this technology.
Data Quality and Availability
For machine learning algorithms to be effective, they require large, high-quality datasets. However, obtaining and annotating such datasets can be resource-intensive. In many industries, decision-makers struggle with insufficient data or data that is not representative of real-world scenarios.
Bias and Fairness
Machine learning models can inadvertently perpetuate biases present in training data. Mitigating bias is crucial to ensuring that algorithms are fair and equitable, especially in sensitive applications like hiring and criminal justice.
Regulatory Compliance
As machine learning applications grow, so does the scrutiny from regulatory bodies. Businesses must navigate complex legal frameworks concerning data privacy, ethical AI use, and transparency. This complexity can lead to hesitancy in adopting machine learning solutions.
The Future of Machine Learning: Trends to Watch
The future of machine learning is bursting with potential. Here are some trends that business leaders and innovators should keep an eye on:
1. Increased Integration with IoT
The Internet of Things (IoT) is generating vast amounts of data, which presents a significant opportunity for machine learning. Integrating ML with IoT devices will enhance analytics, predictive maintenance, and smart city initiatives.
2. Growth of Edge Computing
With the rise of edge computing, machine learning algorithms will increasingly operate on local devices rather than relying on centralized cloud services. This shift will result in faster processing times, reduced latency, and enhanced privacy, making real-time applications more viable.
3. Evolution of Healthcare Applications
Machine learning's potential to revolutionize healthcare continues to expand. From predictive diagnostics to personalized treatment plans, ML is making significant inroads into improving patient outcomes and operational efficiency.
4. Sustainable AI Solutions
The environmental impact of training large machine learning models is a growing concern. As such, there will be a push towards more energy-efficient algorithms and a focus on reducing the carbon footprint associated with AI technologies.
5. Continuous Learning Models
As the business landscape evolves, traditional machine learning models may struggle to keep up. Continuous learning models that adapt to new data in real-time can provide businesses with the agility they need to remain competitive.
Conclusion
The realm of machine learning is advancing rapidly, offering both revolutionary opportunities and significant challenges. Keeping abreast of the latest updates in machine learning not only fosters innovation but also equips businesses with the knowledge to leverage these technologies effectively. As we look to the future, the potential for machine learning to transform industries is immense, paving the way for smarter, more efficient solutions that can truly change the world.
For organizations looking to harness these advancements, staying informed and adapting to the evolving landscape of machine learning is critical. By embracing innovation, businesses can unlock new opportunities and drive substantial growth in an ever-competitive market.
machine learning latest updates